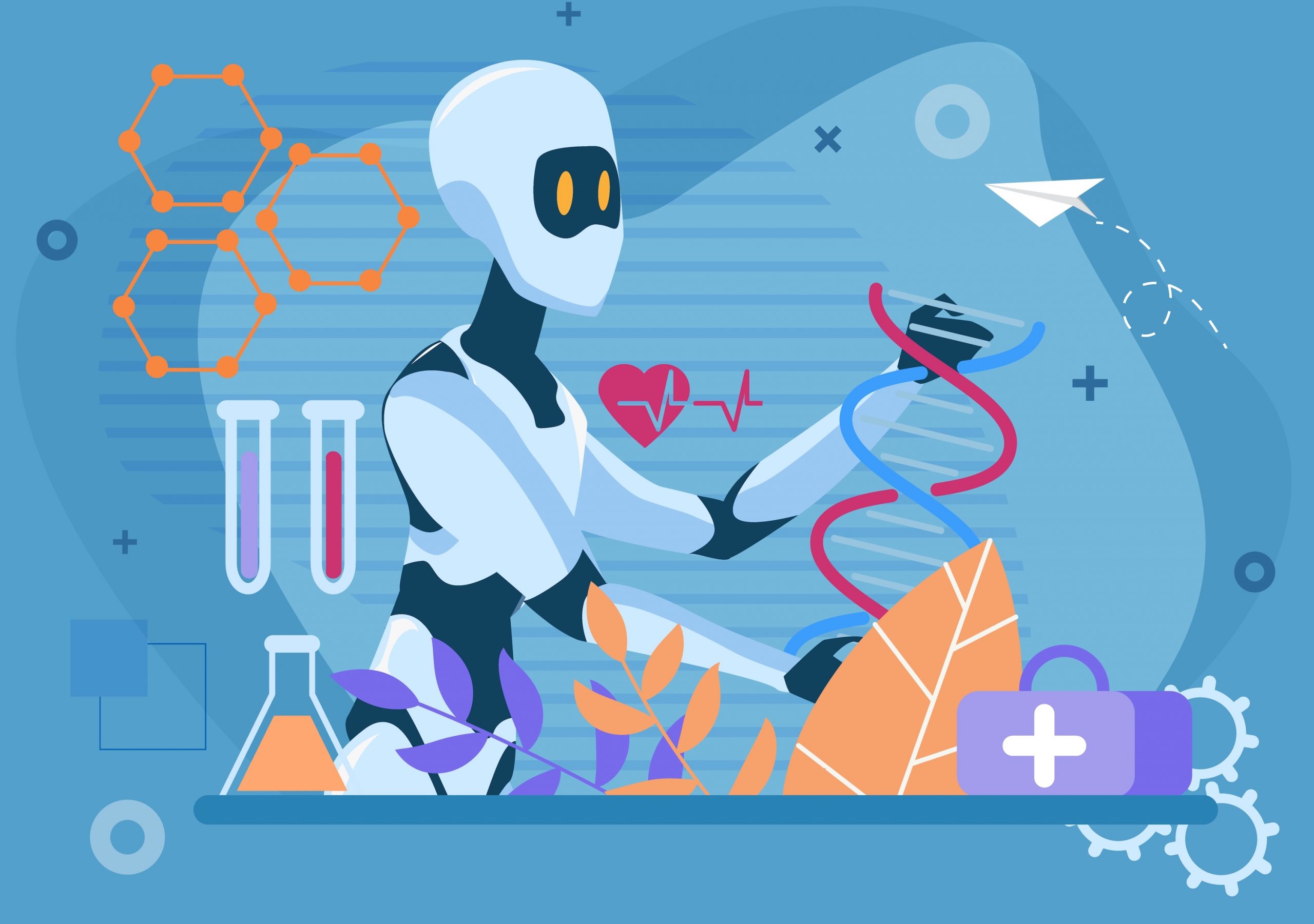
The pandemic has taken the use of intelligent algorithms in the pharmaceutical industry to a new level: the industry needed to radically accelerate the development of new drugs. In the future, the use of artificial intelligence and accumulated data will be an essential part in the fight against rare diseases, in the study of new molecules and in the search for patients.
Author: Irina Efimenko, founder and CEO of Semantic Hub
The background of the issue
The introduction of algorithms for intelligent analysis of big data in the field of pharmaceuticals and medicine began before the emergence of the concept of big data: the first expert systems were created for the use in medicine, which are now more often referred to as decision support systems, such as MYCIN, the development of which began in the 1970s in Stanford University. Since the topic of artificial intelligence (AI) is now quite popular, it seems that all the achievements in this field have been made in recent years, and AI itself is equal to neural networks. However, the theoretical foundations of modern solutions were almost completely laid in the period from 1960s to 1980s.
Nevertheless, the advent of big data and the growth of computing power have brought AI technologies and decision support algorithms to a whole new level. Now there is enough material to feed the algorithms and models, and some solutions have been translated into practice for the first time.
The COVID-19 pandemic played a similar role. It has become a catalyst for the development of AI in medicine, as huge amounts of medical data have been collected around the world and are ready to become a basis for future research.
Drug development
Investments in the development and implementation of an innovative drug can reach $ 3 billion, and the process itself can take up to 14 years. A significant part (45% and more) of the financial and time investment required for the market launch of new drugs is related to the early stages of the drug’s development, including preclinical research. At the same time, not all drugs eventually enter the market. In recent years, pharmaceutical companies have been introducing tools that can accelerate preclinical research and make the development of new therapies more predictable.
According to some estimates, in the next few years, the use of AI can help to save dozens of billions of dollars that are now spent for finding and developing new molecules. That is why the search and development of a candidate molecule (Drug Discovery, DD) is one of the stages where AI is most actively used. The market of intelligent technologies use for the development of molecules is growing very fast. According to Deloitte, it will leap from $160 million in 2018 to $3 billion by 2025 (an average annual growth rate of 52%).
Now the main role of AI is to predict the interaction between molecules, i.e., how the future drug will work with the target in the human body. Artificial intelligence can also be used to study the mechanisms of the disease itself. AI helps to search for new biomarkers and optimize candidate molecules, because on average, out of 10,000 compounds, only one shows a sufficient level of efficiency and safety and successfully reaches the market.
AI also helps in the process of repurposing drugs. In addition, developers of DD solutions create huge databases on existing therapies, targets, and other data, so that biotech representatives can use this information to quickly find answers to complex research questions (one example is geneXplain, founded by a team from Russia). Also, developers take over the processing of all sorts of so-called raw data, which companies can use for their needs.
Another unusual example of a solution focused on the early stages of creating a therapy is the Foldit game. Essentially, it is a crowdsourcing platform where volunteers can contribute to research by experimenting with protein structures. Recently, the game developers have released a new “puzzle” to work with the novel coronavirus. Drug Discovery also includes automated analysis of large volumes of scientific publications. It is also applicable for slightly later stages, for example, for technological scouting or audit and evaluation when a large pharmaceutical company is trying to decide whether to invest in a particular molecule.
There are at least 200 companies now in the world that are looking into the possible use of AI in Drug Discovery: from startups to global corporations of the scale of Microsoft. Dozens of bigpharma companies are implementing the most successful solutions in this area.
The developments that appear these days have both theoretical and applied value, and they transform the industry. In 2019, Exscientia, which was founded by scientists from the University of Oxford, announced a partnership with Roche and also began cooperation with Bayer. Recently, Valo Health has announced the creation of a new platform that stretches the bridge from the DD stage to clinical trials optimization. Insilico Medicine, a Hong Kong-based company with some Russian roots, has announced the discovery of a new target and a potential candidate (new therapy) for idiopathic pulmonary fibrosis, with the possibility of proceeding to the clinical trial stage in record-breaking time.
Another interesting trend is the use of mini-devices, the so-called organ-on-a-chip. These are 3D cellular models in which human biological features can be recreated in vitro. They reproduce the microarchitecture and functions of living human organs such as lungs, intestine, bone marrow, etc. This is a potential alternative to traditional animal testing. This technology was invented by Emulate, a company originally from the Wyss Institute at Harvard University. In 2018, Emulate entered into a partnership with Roche and Takeda. It also signed an agreement on joint research and development with the FDA (US).
Clinical trials
The next area that will soon be transformed by intelligent technologies is clinical research. This is an extremely expensive, long and high-risk process: some clinical trials are never completed. In 2018, 30,000 new entries were made in the registry of clinical trials maintained by the U.S. National Library of Medicine, ClinicalTrials.gov, and the total number of entries made since 2000 is 300,000. However, in 2018, the results were published only for 5,000 trials (less than 35,000 since 2000).
The digital transformation of the field of clinical research has been going on for many years, for example, through the use of Electronic Data Capture solutions. As for the use of AI, it is often focused on technologies related to the analysis of natural language (Natural language processing, NLP, or Natural language understanding, NLU), as well as on Real World Data (RWD).
Over the last few years, there has been an increase in the interest of leading pharmaceutical companies in NLP. One of the most notable developments in this area is Roche’s acquisition of Flatiron, a company that analyzes RWD in oncology with the use of NLP technologies, among others. In clinical trials, NLP and RWD are used to select patients, as well as to generate “comparison data” instead of data for a group of patients who are administered a placebo.
According to some estimates, the world will have accumulated up to 175 zettabytes* of health data by 2025. These arrays can include important information for medicine such as genomic and other omix data, and readings from wearable medical devices. Often it is unstructured textual information, which is one of the most informative data sources but also the one that is difficult to process. This concernes millions of research articles, patents, reports of patient’s own experience of the diagnosis and treatment provided by patients and their friends/family, and this data is of particular value in the case of severe and rare diseases. |
Recently, similar evidence has been published for Duchenne muscular dystrophy. RWD and the “clinical evidence” (Real World Evidence, RWE) which is generated on the basis of their analysis is a special wide-ranging topic. It also transforms the market. According to Deloitte, as early as in 2018, 60% of biopharmaceutical companies used machine learning technologies to analyze RWD, while the rest planned to introduce such solutions in the coming years.
A particularly interesting segment is the search for patients with orphan diseases, including undiagnosed diseases, as well as patients for studies with complex inclusion and exclusion criteria. Semantic Hub implements this search by semantic analysis technologies (understanding the meaning of a text) applied to “Patient Voice.” This concerns big data of the Web 2.0 class, i. e., anonymized patient stories from open sources: social networks, forums, or doctor-patient portals. The analysis of the “patient’s voice” is applicable both to the establishment of a successful strategy for launching innovative therapy, which is sometimes the first of its kind, and to earlier stages, including not only the recruitment of a clinical trial participants but also the definition of its format.
In general, the “patient’s voice” is extremely important for the analysis of the drug’s life cycle stages. It is no coincidence that this type of data is increasingly recognized in the world as a type of RWD. This is stated in the monograph “Studies of Actual Clinical Practice” edited by A. S. Kolbin, which was published in Russia some time ago. Previously, electronic medical records were considered the key type of RWD, but they may be of poor quality. They are undoubtedly a useful source of data for specific directions, for example, on the results of therapy, but some information, including patients’ perception of the therapy, all sorts of aspects of the quality of life and the burden of the disease, is not recorded in electronic medical records and can only be obtained from the patients or their relatives.
The outlines of the future
The awareness of the special role of patients, as well as the importance of RWD, contributes to the increased attention of pharmaceutical companies to AI-based solutions in the B2C segment (i. e., focused on the end user, and in the case of severe diseases, also on their relatives). After all, those who are trying to create breakthrough therapies are focused not only on the “drug path”, but also on the the “patient path”. It is not a coincidence that leading pharmaceutical companies, such as AstraZeneca, Sanofi, Novartis, Janssen, Bayer, and others, are launching their own accelerators in this area. In Russia, a number of such programs have been launched jointly with the Skolkovo Innovation Center.
Often, B2C solutions are focused on supporting diagnostic decision-making. A special type of RWD is generated by personal medical devices, and the development of gadgets is increasingly accelerating this process. As a result of digitalization, any solutions used in clinics, including laboratory tests, functional diagnostics, etc., also become a source of large-scale multimodal medical data. This creates both a challenge for AI developers and new opportunities for research in the field of medicine and pharmaceuticals.
The COVID-19 pandemic has led to a rapid increase in the volume of certain types of data. Information about the quality of life, comorbidity, and some other aspects of the patient experience can become the basis for identifying “uncovered needs,” with the subsequent decision on the creation of a new therapy for a specific patient segment. Patient experience in diagnostics and treatment is the main source of important knowledge for creating a value proposition for a new drug and developing awareness campaigns. Finally, online communication between patients and between patients and doctors can be used to identify signals in the field of pharmacosafety, which, in turn, enables R&D to make valuable discoveries and leads to the early stages of drug development.
In the end, it is the transformation of the patient role and patient-centricity that are the key global trends that can bring pharmaceuticals and healthcare in general to a fundamentally new level.
*Unit of measurement of information, 1 ZByte = 1021 bytes.